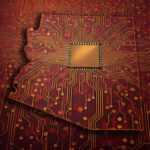
In a class of his own
Dimitri Bertsekas leads innovative reinforcement learning course and research in the Ira A. Fulton Schools of Engineering
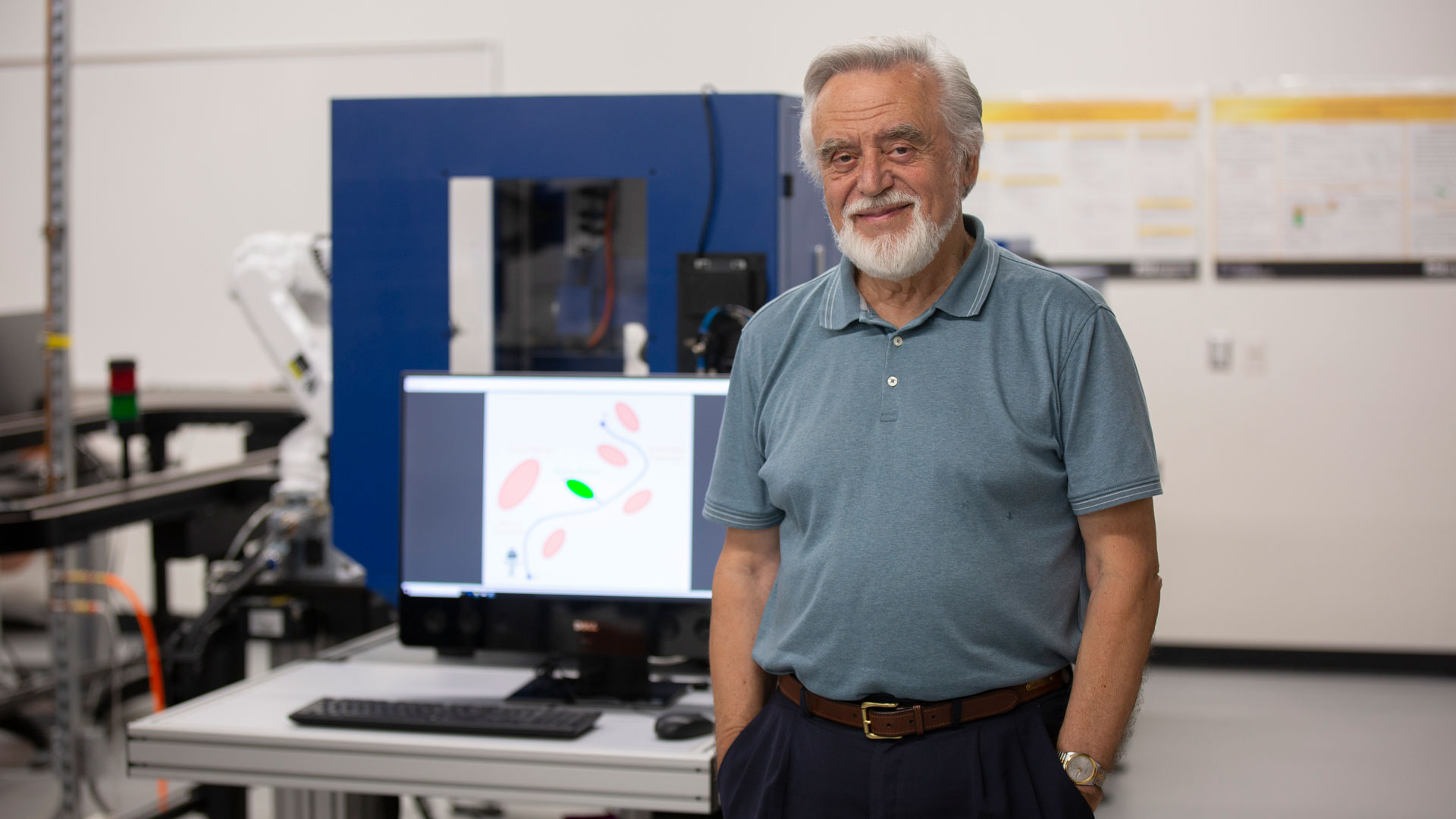
Above: As Fulton Chair of Computation Decision Making in the Ira A. Fulton Schools of Engineering at Arizona State University, Dimitri Bertsekas is leading the establishment of a reinforcement learning research program. The National Academy of Engineering Member is also teaching an updated reinforcement learning graduate course to complement research activities in the field. Photographer: Erika Gronek/ASU
Dimitri Bertsekas’ principal goal since becoming the Fulton Chair of Computational Decision Making in the School of Computing and Augmented Intelligence in 2019 has been to establish a research program in reinforcement learning. This topic is one of the most active areas of artificial intelligence and machine learning that is addressing large, challenging, multistage decision problems applicable to a wide variety of engineering contexts.
The U.S. National Academy of Engineering Member has extensive experience in reinforcement learning and has contributed to research expansion and advancements in the field at the Ira A. Fulton Schools of Engineering at Arizona State University. His research includes multi-robot service systems, RNA folding, structure maintenance scheduling, blood supply replenishment scheduling and economic power generation planning.
Bertsekas also researches artificial intelligence, optimization, linear and nonlinear programming, data communication networks and parallel and distributed computation.
“Easy access to his encyclopedic knowledge of these fields — which has become central to the artificial intelligence revolution — has been a boon to the School of Computing and Augmented Intelligence,” says Subbarao Kambhampati, a professor of computer science, a leading artificial intelligence expert and one of Bertsekas’ colleagues in the school. “It is not surprising that he has been a sought-after member of several large AI-related proposals from ASU.”
Bertsekas is bringing new knowledge to his students through an updated version of his reinforcement learning course, CSE 691: Topics in Reinforcement Learning, which provides the opportunity to initiate graduate-level research in emerging topics across a range of engineering disciplines.
“The new version of the reinforcement learning course in the School of Computing and Augmented Intelligence aims to strengthen the related research activity within the ASU community,” Bertsekas says.
Through this effort, he is providing infrastructure that promotes exploration of the common boundary between optimal control and artificial intelligence that is accessible to students and researchers in both fields.
Bertsekas’ 2021 monograph, “Lessons from AlphaZero for Optimal, Model Predictive, and Adaptive Control,” enhances and supports the design philosophy of his 2020 reinforcement learning book, “Rollout, Policy Iteration, and Distributed Reinforcement Learning,” which serves as the backbone of his new course.
“The 2021 monograph, together with research performed in the challenging and currently very active area of multi-agent systems decision and control, will be used as a major textbook for the 2022 offering of the reinforcement learning class at ASU,” Bertsekas says.
Kambhampati says the course has been an asset to students across the university — and most of his own students have taken the course for credit.
In addition to the reinforcement learning course, Bertsekas teaches research-oriented seminars on the topics of optimization, optimal control, machine learning and artificial intelligence.
“It is humbling to see the seriousness he accords his teaching,” Kambhampati says, adding that Bertsekas writes his books in the areas of reinforcement learning, optimal control and optimization at the rate that many researchers write journal articles.
Videos of Bertsekas’ lectures are available on his YouTube channel, which Kambhampati says has “quite a following among AI researchers around the world.”